Cross–testing Symbolic and Connectionist Machine Learning Approaches in Specialized Acute Appendicitis Databases
Navzkrižno testiranje simboličnih in konektivističnih pristopov strojnemu učenju na specializiranih bazah akutnega vnetja slepiča
Abstract
Purpose: In the world of learning with medical supervised machine approaches, we often face a lack of dataset objects suitable for training a classifier. Two of the most common reasons are the lack of funds to perform all of the required tests and dataset gathering, or simply the condition is too rare to collect a suitable number of cases. In this paper we present the results of a very rare opportunity to test and train classifiers on three acute appendicitis datasets with almost identical structures, but from different sources and of different sizes.
Methods: We performed a parallel variant of cross–testing of two types of classifiers (decision trees [DT] and artificial neural networks [ANN]). Our variant of cross–testing focuses on training the classifiers on one dataset and testing all of the remaining available datasets, including the one derived from the same source, as the training set. To compare the three acute abdominal pain databases of different sizes and origins, we selected 18 parameters from patient medical histories, clinical examinations, and the decision attribute/diagnosis, acute appendicitis.
Results: The comparison of results was based on overall accuracy, sensitivity, specificity, and balance.
Conclusion: The results we obtained were quite surprising, and factors such as dataset size and theoretical power of the methods did not prove to be as important as first expected.
Downloads
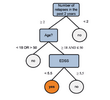